FEDS Notes
April 07, 2017
Measuring Firm-Level Uncertainty
Ample empirical research documents the negative effect of uncertainty on economic activity.2 Unexpected changes in macroeconomic conditions or doubts about the direction of future policy tend to be associated with lower capital investments, reduced hiring, and slower consumer spending.
Encompassing a notion that refers either to an increase in the variance of the distribution of expected outcomes or to a higher probability of more unfavorable outcomes, uncertainty measures discussed in the literature generally consist of two types: time-varying aggregate indicators--such as changes in the dispersion across forecasters' predictions or across firm variables and frequency indexes about economic uncertainty--and the volatility of firm-specific processes.3
Both measures, however, suffer some drawbacks. While time-varying macro indicators might be unable to capture firm-specific shocks that wash out in the aggregate, indexes of volatility rely on cross-sectional variation to identify the effect of uncertainty and, thus, the economic implications might be affected by unobservable differences across agents.
Recent literature has made some progress on firm-level time-varying measures of uncertainty. Hassan et al. (2016) construct a firm-level frequency index of political uncertainty based on filings with the Securities and Exchange Commission; they report that 2/3 of the variation in political risk is idiosyncratic, with the identity of the most exposed firms changing over time and within sectors.
This article proposes an alternative and more general time-varying measure of firm-level uncertainty, the ex post forecast error.4 Drawing on Italian firm-level data--namely, Indagine sulle Imprese Industriali e dei Servizi--, we construct the absolute deviation between an outcome and the firm's past expectation, directly reported in every survey since 1995; the survey collects data on standard balance sheet items (wage bill, employment, sales, investment, capacity utilization, price changes, etc.) and on one-year-ahead expectations of sales, investment, capacity utilization, and price changes. With the expectation reflecting a probability-weighted average across possible outcomes, a deviation between the ex-ante expectation and the actual realization is likely to capture a shift in probability towards outcomes in the tails of the distribution. Such a shift, however, is unlikely to occur as a pure mean-preserving spread—a type of shock also known in the literature as "pure uncertainty."
With this caveat in mind, we construct the ex post forecast error for the capacity utilization rate. As a ratio of firm output to its potential–namely, the extent to which a firm can produce goods, given its current technology and fixed factors of production--capacity utilization tends to reflect the cyclical state of aggregate demand.5 In particular, the correlation between the annualized changes in gross domestic product and the index of capacity utilization is 0.63 for Italy.6
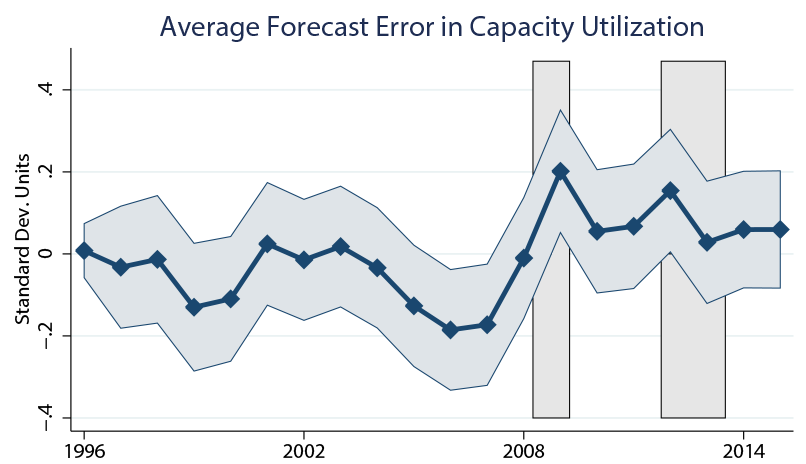
Note: Blue-shaded area represents 95 percent confidence interval; gray bars denote recession periods.
Source: Banca d'Italia, Indagine sulle Imprese Industriali e dei Servizi, 1996-2015.
In a first step, we construct an index of aggregate uncertainty, the average forecast error for the capacity utilization rate, by regressing the firm ex post forecast error in capacity utilization on yearly dummies; figure 1 shows the point estimates and the associated 95-percent confidence interval. For ease of interpretation, we rescaled our index by subtracting its original mean and dividing it by its initial standard deviation. After remaining fairly constant during the late 1990s and early 2000s, the ex post forecast error declined in the mid-2000s, before stepping up in two subsequent periods--first, around the time of the Great Recession and, later, as the European debt crisis heightened. With increases that coincide with the two recent Italian recessions, our index displays the countercyclical behavior typical of uncertainty measures, as documented by Bloom (2014). However, the short available history is an obstacle to a robust evaluation.

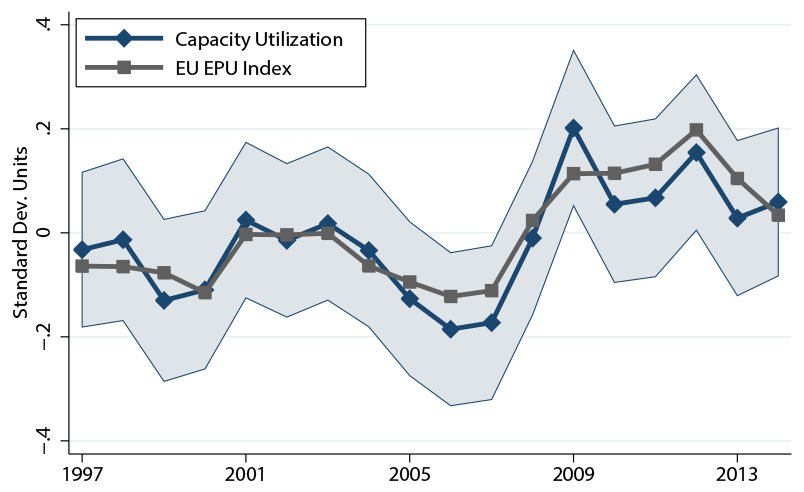
Note: Shaded area represents 95 percent confidence interval.
Source: Banca d'Italia, Indagine sulle Imprese Industriali e dei Servizi, 1997-2014, and Baker, Bloom, and Davis (2016).
Figure 2 compares our index with annual measures of Italian and European policy-related economic uncertainty, developed by Baker, Bloom, and Davis (2016).7 The measures of economic policy uncertainty (EPU) and our index tend to co-move, recording similar increases in uncertainty during the Great Recession and the European debt crisis.8
Table 1. Comparing Measures of Uncertainty: Correlation Table
Avg. CU Error | Italy EPU | Europe EPU | |
---|---|---|---|
Avg. CU Error | 1 | - | - |
Italy EPU | 0.71 | 1 | - |
Europe EPU | 0.89 | 0.79 | 1 |
Avg. CU Error: Average error in capacity utilization forecast.
Italy EPU: Annual average of economic policy uncertainty index for Italy.
Europe EPU: Annual average of economic policy uncertainty index for Europe.
Notes: Correlation matrix across measures of uncertainty, 1997-2014. All correlations are significant at 1 percent.
Source: Banca d'Italia, Indagine sulle Imprese Industriali e dei Servizi, 1997-2014, and Baker, Bloom, and Davis (2016).
Table 1 characterizes more precisely the correlation across the three uncertainty proxies. The average forecast error and the annual version of the Italy EPU index display a correlation of 0.71; the correlation of our measure with the European EPU index is even higher, suggesting a large exposure of Italian firms to uncertainty shocks affecting the whole European market. The correlation between the annual versions of the EPU indexes, which reaches 0.79, confirms a strong link between changes in uncertainty in Italy and Europe.
To investigate whether the ex post forecast error in capacity utilization matters for microeconomic outcomes, we shift our focus to the firm level and propose a model that relates proxies of economic activity to our measure of uncertainty,
$$$\text{ln}\ y_{it} = \alpha + \beta\ CU\ {Error}_{it} + \gamma\ X_{it} + D_{st} + D_i + \varepsilon_{it}$$$,
where $$$y_{it}$$$ denotes firm i economic outcome at time t and $$$CU\ {Error}_{it}$$$ represents the absolute deviation between the expected and the realized capacity utilization rate (in log). We focus on four proxies of economic activity: domestic sales, exports, investment, and employment. In our specifications, we include ex post forecast errors in sales, in employment, and in price changes and proxies for firm size.9 We also add a full set of firm fixed effects and sector-time dummies to control for time-invariant firm characteristics and sector-time-specific shocks. Our model abstracts from the effect of aggregate uncertainty, as estimates of the effect of aggregate sources of uncertainty cannot be separately identified from sector-year-specific shocks.
Our results are summarized in table 2. The forecast error is negatively correlated with all proxies of activity, but its impact is significant only on domestic sales, exports, and employment. An insignificant effect of uncertainty on investment may arise if capital decisions occur before contemporaneous uncertainty shocks materialize--, e.g., if there is a time gap between the order of capital goods and their delivery and the level of uncertainty changes during that time.10 Looking at magnitudes, we find that an increase in the forecast error by one standard deviation (sd) implies a decline in domestic sales of €1014, a decline in exports of €1015, and a decline in employment of 1 worker.11 With an increase in uncertainty of about 0.2 sd during the Great Recession, the change in the forecast error accounts for 1 percent of the total decrease in domestic sales, 1.2 percent of the total decrease in exports, and 1.4 percent of the total decrease in employment.12
Table 2. Contemporaneous Effects of Uncertainty
Variables | (1) ln Salest |
(2) ln Exportst |
(3) ln Invt |
(4) ln Emplt |
---|---|---|---|---|
CU Errort | -0.012*** (0.002) |
-0.013*** (0.004) |
-0.004 (0.007) |
-0.003*** (0.001) |
Firm FE | y | y | y | y |
Sector-Year | y | y | y | y |
Obs. | 17,712 | 17,712 | 17,712 | 17,712 |
R2 | 0.616 | 0.504 | 0.175 | 0.855 |
ln Salest: domestic sales (in log).
ln Exportst: foreign sales (in log).
ln Invt: investments (in log).
ln Emplt: total employment (in log).
CU Errort: forecast error in the capacity utilization rate (in log).
Legend: *** significant at 1%, ** at 5%, * at 10%.
Notes: Firm-level regressions, 1996-2015. Standard errors are clustered at the firm level. All specifications also include age, past domestic sales, past exports, past employment, past investments, past capacity utilization rate, and forecast errors in domestic sales, in exports, in employment, and in price changes.
Source: Banca d'Italia, Indagine sulle Imprese Industriali e dei Servizi, 1996-2015
Next, we look at outcomes in subsequent years to investigate whether the economic effects of uncertainty are persistent; the results are summarized in figure 3, where the number of periods on the x-axis is relative to the year in which the shock occurs, denoted as year zero.
In the year after the shock materializes, higher uncertainty around the forecast of the capacity utilization rate continues to depress domestic sales, exports, and employment; uncertainty has also a significant effect on future investments: a one-standard-deviation increase in uncertainty is associated with a decline in investments of €1023 after one year. While the effect on sales subsides after two periods, uncertainty continues to weigh on investments and employment. After three years, the uncertainty shock mostly dissipates. The effects shown in figure 3 are consistent with patterns of a sharp decline and a quick rebound in economic activity, outlined by Bloom (2009) and documented by Bachmann, Elstner, and Sims (2013) for Germany.
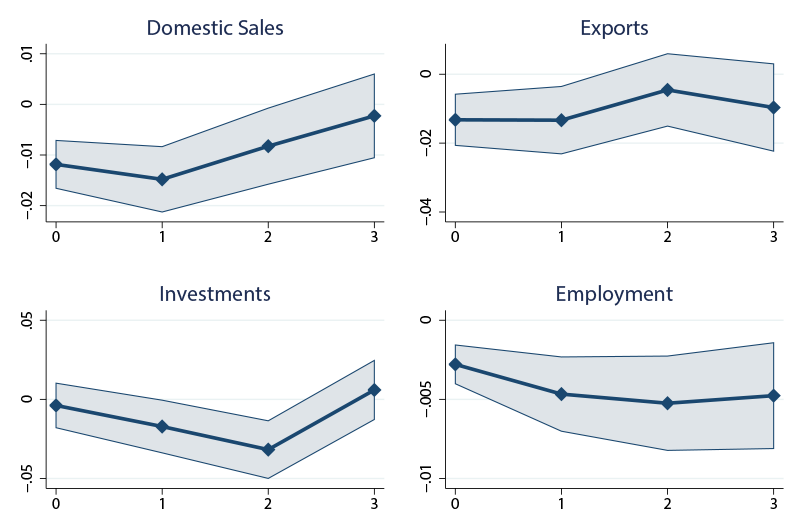
Note: Shaded area represents 95 percent confidence interval.
Source: Banca d'Italia, Indagine sulle Imprese Industriali e dei Servizi, 1996-2015.
Our findings confirm that the firm-level uncertainty around the capacity utilization rate is negatively associated with indicators of economic activity. However, even looking at the cumulative impact, firms adjust their sales, investment, and hiring plans very little in response to changes in firm-specific uncertainty, as in Arlsan et al. (2015). The small magnitude of the effects may be related, in part, to the availability of data at a yearly frequency: positive and negative uncertainty shocks might cancel out over a year. Collecting higher frequency firm-level data on the uncertainty surrounding the capacity utilization rate might be an important step toward improving our understanding of idiosyncratic uncertainty shocks.
References
Arslan, Yavuz, Aslıhan Atabek, Timur Hulagu, and Saygın Sahinöz. (2015). "Expectation errors, Uncertainty, and Economic Activity," Oxford Economic Papers, 67(3): 634–66.
Bachmann, Rüdiger, Steffen Elstner, and Eric R. Sims. (2013) "Uncertainty and Economic Activity: Evidence from Business Survey Data," American Economic Journal: Macroeconomics, 5(2): 217-249.
Baker, Scott R., Nicholas Bloom, and Steven J. Davis. (2016). "Measuring Economic Policy uncertainty," Quarterly Journal of Economics, 131(4): 1593-1636.
Banca d'Italia, Indagine sulle Imprese Industriali e dei Servizi, 1996-2015.
Bloom, Nicholas. (2009). "The Impact of Uncertainty Shocks," Econometrica, 77(3): 623-685.
Bloom, Nicholas. (2014). "Fluctuations in Uncertainty," Journal of Economic Perspectives 28(2): 153-175.
Corrado, Carol, and Joe Mattey. (1997). "Capacity Utilization," Journal of Economic Perspectives, 11(1): 157-167.
Federal Reserve Bank of St. Louis (2017). FRED Economic Data, OECD series LORSGPORITQ659S and BSCURT02ITQ160S, https://fred.stlouisfed.org/series (accessed on March 1, 2017).
Guiso, Luigi, and Giuseppe Parigi. (1999). "Investment and Demand Uncertainty," Quarterly Journal of Economics, 114(1): 185-227.
Hassan, Tarek A., Stephan Hollander, Laurence van Lent, and Ahmed Tahoun. (2016). "Aggregate and Idiosyncratic Political Risk: Measurement and Effects," Available at SSRN: http://dx.doi.org/10.2139/ssrn.2838644 .
Jurado, Kyle, Sydney C. Ludvigson, and Serena Ng. (2015). "Measuring Uncertainty," American Economic Review, 105(3): 1177-1216.
Kydland, Finn E., and Edward C. Prescott. (1982). "Time to build and aggregate fluctuations," Econometrica, 50(6): 1345-1370.
Leahy, John V., and Toni M. Whited. (1996). "The Effect of Uncertainty on Investment: Some Stylized Facts," Journal of Money, Credit and Banking 28(1): 64-83.
Scotti, Chiara (2016). "Surprise and Uncertainty Indexes: Real-Time Aggregation of Real-Activity Macro Surprises," Journal of Monetary Economics, vol. 82, pp. 1-19.
1. Federal Reserve Board, 20th Street and Constitution Ave NW, 20551, Washington, DC. Contact: [email protected]. I would like to thank Norm Morin for his insightful comments. Data for graphs and regression results for this project were obtained through the Bank of Italy Remote access to micro Data (BIRD). The views expressed in the article are those of the author and do not necessarily reflect those of the Federal Reserve System or of the Bank of Italy. Return to text
2. For a recent literature review, see Bloom (2014). Return to text
3. Among aggregate indicators, Bachmann, Elstner, and Sims (2013) propose the variance of forecasters' expectations; Jurado, Ludvigson, and Ng (2015) extract the common components of forecast errors for several macroeconomic time series; Baker, Bloom, and Davis (2016) use the frequency of news related to policy uncertainty; Scotti (2016) exploits the forecast error between the Bloomberg median expectation and the release of real activity indicators. Other studies, such as Leahy and Whited (1996) and Bloom (2009), use stock market volatility. Guiso and Parigi (1999) calculate the variance of the subjective probability distribution of demand forecasts; their data allow them to exploit only the cross-sectional variation in demand uncertainty. Return to text
4. Bachmann, Elstner, and Sims (2013) use the average size of the forecast error as a proxy of uncertainty and show that it is positively correlated with the dispersion across the ex-ante disagreement and with the ex post distribution of errors, other traditional measures of uncertainty. Return to text
5. See Corrado and Mattey (1997) for a more detailed discussion of capacity utilization. Return to text
6. Data for Italy were retrieved from Federal Reserve Bank of St. Louis (2017). Return to text
7. The country-level indexes are based on newspaper articles regarding policy uncertainty. The authors draw on Corriere della Sera and La Repubblica for Italy; the European index combines French, German, Italian, Spanish, and U.K. indexes. For more details on the methodology, see Baker, Bloom, and Davis (2016). The data are available on the Economic Policy Uncertainty website, at http://www.policyuncertainty.com/europe_monthly.html. Return to text
8. We rescaled the EPU indexes by the dispersion in the capacity utilization forecast error. Return to text
9. Ex post forecast errors for sales, employment, and price changes are not good indicators of business cycle activities and their empirical properties are not consistent with other proxies of uncertainty. Return to text
10. The time gap is commonly known as time to build in the literature. See, for example, Kydland and Prescott (1982). Return to text
11. The effects correspond to a decline in domestic sales of 0.86 percent of a sd, a decline in exports of 0.66 percent of a sd, and a decline in employment of 0.24 percent of a sd. Return to text
12. Between 2008 and 2009, total domestic sales declined 24 percent, total exports 22 percent, total investment 22 percent, and total employment 4 percent. Return to text
Tito, Maria D. (2017). "Measuring Firm-Level Uncertainty," FEDS Notes. Washington: Board of Governors of the Federal Reserve System, April 7, 2017, https://doi.org/10.17016/2380-7172.1966.
Disclaimer: FEDS Notes are articles in which Board staff offer their own views and present analysis on a range of topics in economics and finance. These articles are shorter and less technically oriented than FEDS Working Papers and IFDP papers.